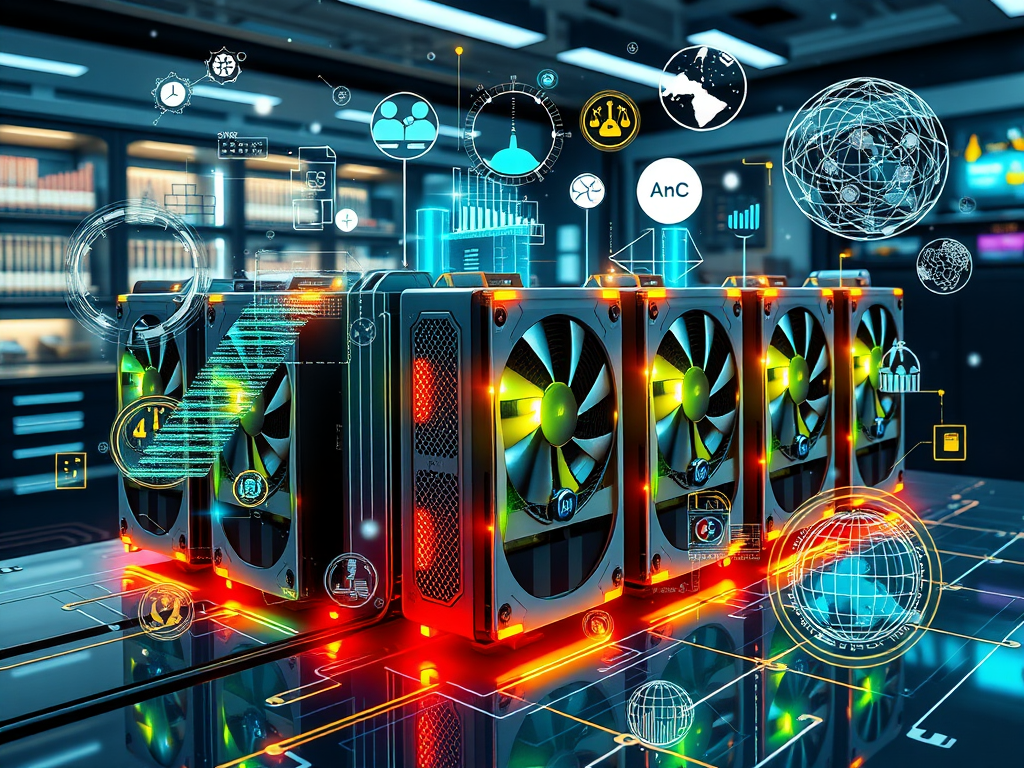
Graphics Processing Units (GPUs) have transformed from tools for gaming graphics to essential components in scientific research. GPU clusters are collections of connected GPUs that work together to perform complex calculations. This technology has opened new doors in various fields of study, enhancing our ability to analyze data and simulate systems. Here are several important use cases for GPU clusters in scientific research.
1. Molecular Dynamics Simulations
Molecular dynamics (MD) simulations are crucial for understanding how molecules behave over time. This is particularly important in biochemistry, drug discovery, and materials science.
How It Works
Researchers use MD simulations to study interactions between molecules, such as how proteins fold or how drugs bind to their targets. These simulations require a lot of calculations, as they track the positions and movements of thousands of atoms over time. GPU clusters significantly speed up these processes by handling many calculations simultaneously.
Real-World Applications
For example, in drug discovery, scientists can simulate how a new drug interacts with a protein associated with a disease. This helps identify promising candidates for further testing, reducing the time and cost of bringing new medications to market.
2. Genomics and Bioinformatics
In genomics, scientists analyze vast datasets generated from DNA sequencing. This field is critical for understanding genetics and personalized medicine.
The Role of GPU Clusters
GPU clusters enable faster processing of genomic data, which can include millions of DNA sequences. They help speed up tasks like genome assembly, variant calling, and gene expression analysis.
Example Tools
Tools such as GATK (Genome Analysis Toolkit) leverage GPU acceleration to process genomic data more efficiently. This allows researchers to quickly identify genetic variations linked to diseases, helping in the development of targeted therapies.
3. Climate Modeling
Climate models are complex simulations that predict future climate conditions based on various environmental factors.
Importance of GPU Clusters
These models require significant computational power to integrate data from different sources, such as atmospheric, oceanic, and land data. GPU clusters excel in performing the massive calculations needed for these models, enabling scientists to make more accurate predictions about climate change and its impacts.
Impact on Policy and Planning
By providing better predictions, researchers can inform policymakers about potential climate scenarios, helping them create effective strategies for mitigation and adaptation to climate change.
4. Artificial Intelligence and Machine Learning
Machine learning (ML) and deep learning (DL) are increasingly used in scientific research to analyze complex datasets and identify patterns.
Benefits of GPU Acceleration
GPU clusters are particularly suited for training deep learning models, which require processing large amounts of data. The parallel processing capabilities of GPUs allow researchers to train models much faster than traditional CPUs.
Applications Across Disciplines
In fields such as astrophysics, researchers use ML to analyze data from telescopes, identifying new celestial objects. In healthcare, AI algorithms can help in diagnosing diseases from medical images, improving patient outcomes.
5. Computational Fluid Dynamics
Computational fluid dynamics (CFD) is the study of how fluids move and interact with surfaces. This field is important in engineering, environmental science, and physics.
Role of GPU Clusters
GPU clusters accelerate the complex calculations needed for CFD simulations. These simulations can model airflow around aircraft, water flow in rivers, or even blood flow in arteries.
Practical Implications
The insights gained from these simulations can inform the design of more efficient aircraft, improve flood management strategies, and enhance medical treatments through better understanding of blood flow dynamics.
6. High-Energy Physics
Experiments in high-energy physics, such as those conducted at CERN, generate vast amounts of data from particle collisions.
Data Processing Challenges
Analyzing this data is challenging due to its sheer volume and complexity. GPU clusters help manage and analyze this data efficiently, allowing scientists to simulate particle interactions and study fundamental questions about the universe.
Discoveries and Innovations
Using GPU technology, researchers can explore phenomena such as the Higgs boson or dark matter, leading to significant advancements in our understanding of physics.
7. Neuroscience and Brain Modeling
Understanding the human brain is one of the greatest challenges in science. Neuroscience research relies on simulations of brain activity and neural networks.
Contributions of GPU Clusters
GPU clusters facilitate large-scale simulations that can model complex brain processes. These simulations help researchers understand how neurons interact and how brain activity correlates with behavior.
Implications for Health
Insights gained from these models can lead to better treatments for neurological disorders such as Alzheimer’s and Parkinson’s disease, ultimately improving patient care.
Conclusion
GPU clusters are revolutionizing scientific research by providing immense computational power for a variety of applications. From simulating molecular interactions to predicting climate change, these technologies enable scientists to analyze large datasets quickly and efficiently. As research continues to evolve, GPU clusters will play an increasingly central role in driving innovation and discovery across multiple scientific fields. Their ability to handle complex calculations and massive datasets will continue to unlock new possibilities, helping researchers tackle some of the world’s most pressing challenges.